Severence M. MacLaughlin, Ph.D
Managing Partner
DeLorean Artificial Intelligence
Artificial Intelligence, Big Data, Data Science… are all buzzwords that inspire an idyllic future of smooth, efficient operations and offer a panacea for many ills plaguing an industry. This is no different for healthcare where, over the past four to five years, numerous articles have detailed what could be for payers, providers, and other associated industries. I am just as guilty in perpetuating this hope and desire through authoring articles in 2018 and 2019 touting this very thing. Sadly, the hype of artificial intelligence has not come to fruition and little has happened in the way of progress for healthcare. AI was supposed to decrease costs and increase health outcomes. One specific industry analysis in 2017 predicted that the
economic impact of AI on the global economy would translate into approximately $15T and of that $938M would hit the North American healthcare industry. It was not just that efficiencies would be gained, but
actually improved health of patients would be generated as well.
Recently I reviewed the body of literature for AI implementations in the healthcare payer market looking for any demonstrable result. Though I found myself back in 2017 when, after sequential market love trysts
with Big Data and Data Science, AI-first earned its enigmatic status and people were left wondering many things; could these terms be interchanged, is one a rose and the other a mysterious flower that smelled
as sweet? A Shakespearean comedy or tragedy? AI was set to transform the world but three years later the world, particularly healthcare, is still waiting.
The high-impact predictions of AI on healthcare are perpetuated by some of the biggest names in the industry such as McKinsey and Gartner. These organizations use the typical examples of how AI, or as I would term them, advanced analytics, increased efficiency in claims processing or adjudication/cost recovery. These examples clearly demonstrate the support of bureaucratic processes and reducing costs associated with administering healthcare services, but they have nothing to do with improving health
itself. Go figure. My favorite red herring used as an example of how AI is advancing is of drug discovery in the pharmaceutical industry. Though, for the past twenty years that I have participated in the healthcare, life sciences, and the data/advanced analytics/AI industries I have not seen any progress, whatsoever, even in this regard. Pharma and healthcare payers alike suffer from the same problems; a slowness to act in adopting more advanced technologies compounded by issues of implementation and scaling. One point of observable excellence is that these large organizations and bureaucracies are good at seed funding small AI, or advanced analytics, projects that turn out to be what I refer to as a ‘science project’. That is
to say, these initiatives are siloed, vertical-specific, and do not realize an ROI or cannot be implemented to scale at an enterprise level. There has also been significant damage to the advanced analytics and AI movement in the healthcare marketplace by some of its biggest players, AI personas that were marketed well but when it came to deliverables, clearly failed. In healthcare, IBM Watson is the poster child for over-promising and under-delivering. I give IBM Watson and the marketing department behind it kudos. My mother, a technology Luddite, had even heard of Watson. What she thought is that Watson was AI and that it arrived in a cube that was then plugged into a client or organization’s computers and voila a sentient AI was uploaded. The marketing was clearly excellent. The healthcare market itself was ready for such a solution and readily bought Watson. What unfolded was a number of disasters, MD Anderson is the highest-profile. The good news is that the IBM Watson marketing juggernaut helped the layman understand that AI could do great things from business to healthcare, however, the bad news is that IBM Watson may have spoiled
the market for others – making buyers a bit gun shy. One clear success story that should be noted, as well as applauded, is the utilization of computer vision applications for radiology and other medical imagery assisted diagnostics. Phillips Healthcare won the race to achieve FDA 510(k) approval in 2019 for their technology, which was quickly followed by others. One of the most recent techs being Zebra Medical Vision which came out this past July. This is a key area where the literature can point to a time when AI actually advanced health outcomes for patients, decreased
costs, and conveyed a clear path for enterprise-wide adoption. In my opinion, this AI will help radiologists and pathologists enhance their diagnostic capabilities with the ability to recognize pathophysiologies at
stages previously unable to do so. Other notable AI highlights are Quantx, Apple Watch ECG, Aidoc, and EchoMD AutoEF. What do all of these success stories have in common? The developers and technology had access to large data sets that could be used for training and testing the computer vision AI applications, ergo – these organizations had their data act together so to speak. This data needed to be processed, stored, accessible, and useable.
You might think this is a given, however, even with impediments such as slow adoption and scaling issues, the largest challenge for healthcare and life sciences organizations to overcome to achieve parity with
respect to the adoption of advanced technologies such as AI when compared to other commercial sectors is a structured, cohesive data environment. For AI to be successful in an organization, it must crawl before it walks and walks before it runs in terms of data.
Data is the key to the success of any artificial intelligence and its implementation. Where data comes from is numerous; structured, unstructured, sourced from within a company or externally. In healthcare,
data is captured in a number of verticals: Member, Patient, Physician, Credentialing, Care Management, Claims, Health Outcomes, Sales, Enrollment, Benefit Configuration, Audit Compliance, Contract Review,
and Operations. It could also include sourcing data from legacy systems, post-merger integrations, or different operating or cloud platforms. This is even before bringing in the data available through Electronic
Health Records (EHR). The Clinton and Obama administrations advanced the cause to implement a consistent EHR, driving and mandating “meaningful use” of EHRs by 2014. With the progress of EHRs, I am excited to see the development of Personal Health Records (PHRs) and how blockchain technologies may make the portability of this data the new norm. I would direct you to a fantastic review article by R.S.
Evans (2016), “Electronic Health Records: Then, Now and in the Future” for more information around the history and evolution of EHRs. This is a wealth of data, which is collocated in one environment would act to enhance the informed decision capabilities of the HC payer. Data needs an environment to live and thrive. It needs to be organized, accessible, useable, and portable. DeLorean AI calls this being in control of your Data Lifecycle Value Chain (see Figure 1). Most companies are not in control of this. If you spoke to any data engineer, analyst, or scientist they would tell you that if an entity’s data is controlled the outputs from advanced analytics, data science, and/or AI are GOLD. It is well worth the investment to design a data ecosystem that can both coordinate and harness an
organization’s data that operates under a Single Source of Truth or One True Source.
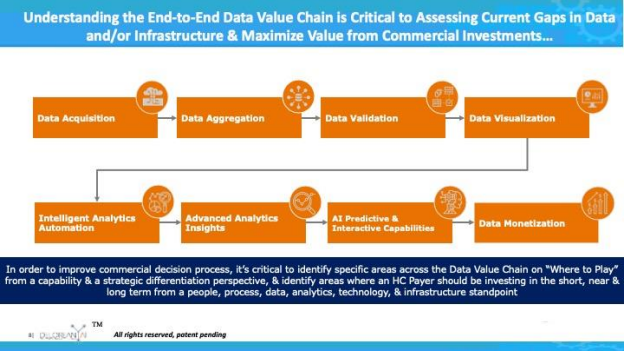
Figure 1: Data Life Cycle Value Chain – being in control of an organization’s data allows for predictive and interventive artificial intelligence. This should be done with the bevy of healthcare data. However, this presents more unique issues. There are challenges associated with migrating and collocating available data into one environment to generate the One True Source. Complications for HC payer organizations arise due to legacy systems, lacking internal capacity, needed upskilling, buy-in from the C-suite to ensure a clear digital vision, and multiple platforms in use due to lack of foresight in planning and execution of a unified IT or digital transformation. It is hard to fault the CIO or the organization here, as it is common for HC payers to buy multiple data and digital products without fully understanding the interoperability or defining the ultimate end game. The end game is to utilize this data to inform decisions, increase health outcomes, decrease costs, and enhance regulatory compliance. Interestingly, one of the greatest obstacles to deriving true value from
the country’s medical and healthcare data is how disparate the sources are in a single organization. With respect to population health the inability of our healthcare system to source data for meaningful analysis whether that be by the Department of Health and Human Services, NIH or CDC is a clear challenge and obstacle to generating the appropriate medical response in times of need, the Covid-19 pandemic is a good example, as well as understanding disease onset, progression, and mitigation. Compounding these obstacles within the US healthcare system are the four distinct silos of constructive data that could be used to better understand pathophysiologies and their respective etiologies: Provider, Payer, Pharmacy, and Pharma (please refer to Figure 2). In countries where socialized medicine is the main source of healthcare, patient data and information is more centralized within one organization. This is not to say these organizations have achieved a Single Source of Truth healthcare data repository. However,
they are a few steps closer to achieving the end goal when compared to the US. In the US, the most valuable data is found in the HC payer’s possession.
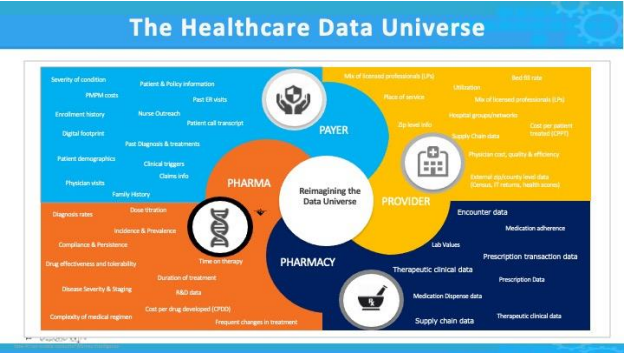
Figure 2: The Healthcare Data Universe divided into four categories: Provider, Payer, Pharmacy & Pharma. There are over 120 HC payer organizations within the US ranging from behemoths such as Aetna, United
Healthcare, and Anthem to the smaller local Blues and institutions. This is where the treasure is. Why? A provider may have data for thousands, but some payers have access to the same data for MILLIONS. Any good scientist will tell you that if there is an “n” of millions the results of an analysis will be much more precise – if you will, the trove is the healthcare payer’s data. The kingdom is the longitudinal tracking of patients and disease/onset/mitigation/treatment. The castle is the ability to bring disparate sources of data together that describe a single member on a scale of millions of ‘n’. The keys, however, are AI and data science. We have discussed the endless challenges and obstacles associated with accessing the answers that lay in these HC Payer data troves, and they are significant. But why has artificial intelligence, data science, and other advanced analytics not yet been applied to generate the desired increased health outcomes,
decreased costs, and associated enhanced regulatory compliance? We have the methodology, the tools, and the data sources. There are some companies making significant progress helping clients organize their data in a methodical way such as having a system organized around a One True Source environment. One example is Cognizant Technology Solutions, specifically developing their Adaptive Data Foundation (ADF) to bring in multiple data sources, organize them, and provide healthcare-specific analytics. Cognizant’s opportunity is when they are able to cross-pollinate ADF with their product, TriZetto, and the nascent data encompassed in its
claims processing. When this happens, they will be a force to be reckoned with. Another strong player in the healthcare payer space is Teradata’s Vantage platform, with strengths in data organization and advanced
analytics combined with CPU strength and speed. One of DeLorean AI’s junior data scientists is quoted as saying, “Wow, this platform is powerful, intuitive, and fast… Can we use Vantage for all our work?”. Finally, Lumiata has a series of products that look at the entire lifecycle of healthcare data. Specifically, I have been impressed with their Healthcare Data Management products that unite disparate data sources and bring order to chaos, further allowing faster model building with the ultimate result of decreasing
risk and cost. DeLorean AI believes that the answer to achieving the end goal and deriving real health outcome results is straight forward and requires:
• A single, cohesive data environment
• A true understanding of data sourcing, usability, & accessibility
• The ability to formulate appropriate AI models
• Possessing the right skillsets, both medical and technical
• Clear vision of the bigger picture
• Unifying leadership that creates buy-in and can drive efforts
Back to Shakespeare – a rose by any other name…. The hype of artificial intelligence has caused a “muddying” of the waters of the analytics world of what is analytics, advanced analytics, data sciences, or artificial intelligence. Leaders, practitioners, vendors, and client companies have started interchanging these terms. Words and definitions have meaning. Analytics are not the same as AI, however, they are used interchangeably intentionally or not. My favorite anecdote of interchanging analytics and AI comes from when I worked for a large Fortune 500. At the end of 2017, we supposedly had 400 data scientists globally. Then beginning in January 2018, all of a sudden we had 5,000 AI specialists. Did we go on a hiring spree? No, the company just changed the titles of anyone who had the word ‘analyst’ in their title to an AI specialist. I have a colleague and dear
friend that has a potent test to see if the application, pitch, or technology is actually AI. He replaces the term ‘artificial intelligence’ with ‘analytics’, if the meaning does not change then inevitably the description, pitch or technology is basically analytics dressed up for marketing purposes. However, if the meaning does change then there might be some AI power there. The US healthcare payer market NEEDS the implementation of a true AI system that is capable of sourcing multiple data sources (structured and unstructured) to look forward and derive predictions, and NOT backwards with analyses alone, to give payers, providers and patients better choices in care that reflect outcome, cost, and regulatory compliance. DeLorean Artificial Intelligence has developed a patent-pending technology, Real-Time Medical Care Management AI (RTMCM AI) that meets those needs. Notably, RTMCM AI is a System of Intelligence (SOI) that develops mathematical profiles of patient journeys and treatment methodologies for specific pathophysiology such as COPD by sourcing information from claims, pharmacy, physician data, and external sources. The RTMCM AI Model can:
Facilitate Predictions:
- Real-Time Member Health Directionality based on primary diagnosis code
- Member Health Trajectory (outcome & cost)
- Disease Onset Management
- Care Gap Analysis
- Terminal Disease Journey Prediction & Mitigation
- Drug Adherence Driver Analysis
- Forecasted Cost & Utilization
- Member Level Cost & Utilization
Provide Intervention:
A. Next Best Care Action
B. Next Best Healthcare Provider Action
C. Provider Intervention Recommender
D. Medical Adherence
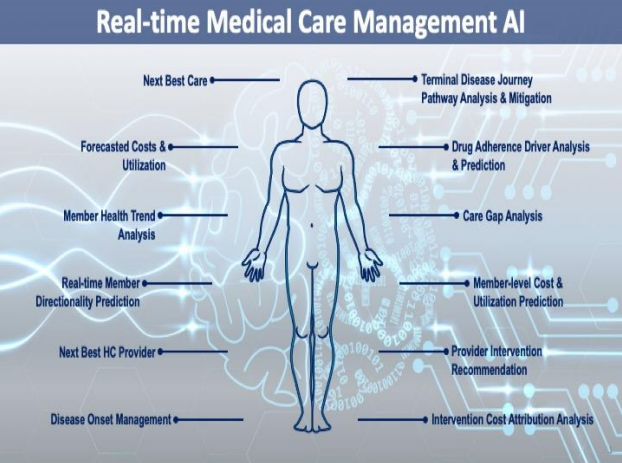
Figure 3: Real-Time Medical Care Management Artificial Intelligence (RTMCM AI) illustrated predictive and interventive AI outcomes. RTMCM AI proprietary technology provides real-time predictions and interventive next best actions for care managers and medical directors to drive the most efficient treatment modalities and better health outcomes. RTMCM AI decreases per member per month (PMPM) costs as well as decreases the medical loss ratio (MLR). DeLorean AI’s clients have decreased their PMPM for the sickest 1% by $100 ($1200 annualized) and on average saw a $10 PMPM decrease ($120 annualized) for the other 99% of members. RTMCM AI has been implemented in major disease state management such as Cardiovascular, COPD, Diabetes, and Juvenile Asthma.
Please join us on our journey……